As a major part of their product development process, Valtra needed to cut field costs related to tractor warranty claims. Advian responded with a Machine Learning solution that gives insight to which tractor parts are at a high risk of failing more often in the future. The solution collects data from all over, for example field data from sensors on the tractors stored in AWS, and data from authorised repair shops to produce a prediction of warranty costs for each tractor part. The final result enables Valtra to identify critical areas of improvement in their product development process, cut costs, and provide quality services to their customers.
Valtra is an agricultural machinery manufacturer based in Äänekoski, Finland, and the leading tractor manufacturer in the Nordics. They have a long history of nearly 100 years, and their tractors and services are recognised for their reliability, versatility, and durability.
Valtra's foremost need was to improve their product development process to be able to cut field costs related to tractor warranty claims. To meet the need, Advian's team delivered a solution that estimates the probability of tractor parts failing in the remaining warranty period as well as produces a forecast of future warranty costs for each individual tractor part. The solution functions as a vital source of information for Valtra to clarify the focus areas of their product development process.
Additionally, Advian's solution brings new possibilities for developing predictive maintenance. The earlier Valtra notices a trend of certain tractor parts failing more often or identifies parts that have high future estimated warranty costs, the sooner they can take action. For example, calling tractors for maintenance, creating individualised maintenance plans, or rebooting manufacturing processes to avoid any major break-downs.
"Working together with Advian has been smooth and straightforward, leaving the customer feeling good. Also, the operations are very professional. With Advian's experts, we are progressing and achieving great results. Flexibility according to the customer's needs has also been a good characteristic of this team." – Lasse Valkonen, Field Quality Lead, Valtra
Estimating with Machine Learning
The challenge in estimating probabilities and producing forecasts was to find a solution that gathers the huge amount of dispersed data and discovers correlations between mechanical failures and the conditions where the failures occur. The approach best suited for this was a Machine Learning -based solution that entailed different techniques and steps depending on how common the mechanical failure is. Matti Karppanen, Advian's Senior Data Scientist and key member in the project, talks more about the solution:
“Our goal was to find correlations between the conditions where tractors fail and due to which parts. We used IoT sensor data, also called stress data accessible in AWS, collected every five seconds by the sensors on the tractors, which tells that the amount of data at hand was huge. We also got to use warranty claim data and, as my colleague likes to call them, "the birth certificates of tractors"”, says Matti.
To manage the large volumes of data and to forecast the probabilities of tractor failures, the solution included the use of XGBoost (eXtreme Gradient Boosting), which is a Machine Learning library, and Weibull distribution model.
“The main idea and output of the solution is a survival graph that shows the probability of the tractor not failing as a function of time. We then drill down to further layers that represents the probabilities of the tractor failing due to different reasons, such as transmission failure, engine failure, cabin failure, and electronics failure. In theory, we could drill down to the last screw of the tractor", Matti explains.
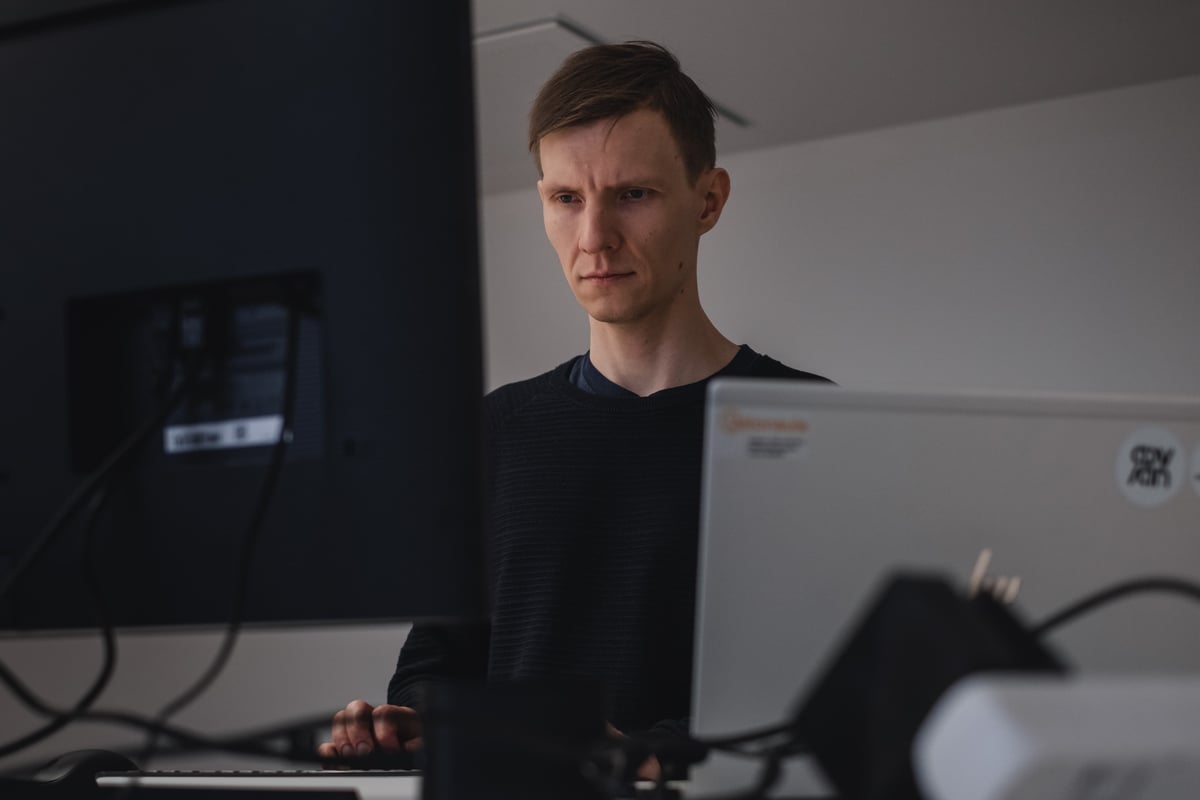
However, the final model wasn't the most obvious choice for executing the application. After a lot of testing, research, exploring of machine learning libraries and other research papers, the team decided to kill the first model and go with the final, perhaps a bit more complex, approach:
“The biggest challenge that we faced was the first model we tried for generating the survival graphs. The results that the model produced were pretty wild. Still, we thought it was the simplest way of doing and wanted to stick with the original model. At the end, after tons of research, we managed to come up with another model that wasn't that straightforward, but gave good results. Sometimes it's for the best to kill your first idea and move on," Matti concludes.
The final result of Advian's Machine Learning application is a dashboard, where a person from Valtra can choose a time span to see the estimated warranty costs for each tractor part in the future. The dashboard gives indication to what parts will be more costly in the future and what kind of parts are at a high risk of failure in specific circumstances – enabling Valtra to act accordingly without a delay.
Developing predictive maintenance and on-time decision making
The dashboard provides Valtra with early warning signs about unreliable parts and tractor subsystems, and a part-by-part prediction of warranty costs, which is a crucial part of their product development. This also paves the way for developing predictive maintenance in form of creating individualised plans during mandatory check-ups and switch any parts that are more likely to fail in the near future.
Not only is the new solution cost-effective, but also helping Valtra provide quality services to their customers all over the world.
Topics: Ai, News, Advanced Analytics, Machine Learning